مقدمة
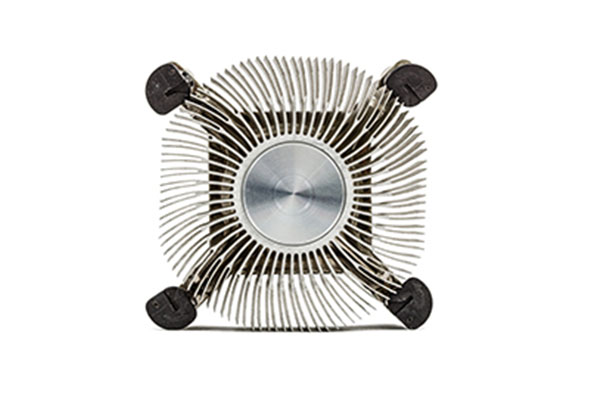
A GPU cooler is a thermal management system designed specifically for graphics processing units, capable of effectively reducing the heat generated during high-performance computing. With the rapid advancement of AI technologies, GPU coolers have become key components in data centers and server infrastructures. In this article, we’ll cover the importance of GPU cooling, how it works, and its role in the AI era—helping you understand why efficient cooling is critical to today’s computing environments.
جدول المحتويات
- Why Has GPU Cooling Become So Important in the AI Era?
- How Do GPU Coolers Work?
- How Do Data Centers Tackle AI Server Cooling Challenges?
- Market Trends in High-Performance GPU Cooling Solutions
- How to Choose the Right GPU Cooling System for AI Workloads
- التعليمات
1. Why Has GPU Cooling Become So Important in the AI Era?
AI is transforming the computing landscape. As businesses and research institutions race to train and deploy increasingly complex AI models, demand for high-performance computing hardware is skyrocketing. At the heart of this trend is the widespread use of GPUs.
The Issue: Training and inference of AI models require massive parallel computing power, pushing GPUs to run at high loads for extended periods.
Here’s the kicker: today’s AI workloads force GPUs to run near maximum performance continuously, generating much more heat than traditional computing tasks. Take high-end GPUs like NVIDIA’s A100 or H100—these can have TDPs (Thermal Design Power) between 300 and 700 watts, and often run close to full load for days or even weeks during intense training sessions.
The Risk: Inadequate cooling leads to serious problems. High temperatures reduce performance (thermal throttling), shorten hardware lifespan, or cause permanent damage. Given that high-end GPUs can cost tens of thousands of dollars, this risk is one that businesses can’t afford.
Worse yet, in high-density server environments, one poorly cooled GPU can raise the temperature of the entire system, triggering a domino effect across nearby components. In the worst-case scenario, this can destabilize the system or cause full-scale failure—resulting in costly downtime and data loss.
The Solution: Efficient GPU cooling systems are now vital infrastructure in AI computing. Modern GPU cooling isn’t just about heatsinks—it involves advanced materials science, fluid dynamics, and precision engineering to keep these computing powerhouses stable at optimal temperatures.
Impact of AI Workloads on GPU Cooling |
---|
عامل |
—————— |
Usage Duration |
Utilization Rate |
Heat Output |
Cooling Needs |
AI-Driven GPU Market Growth
As AI adoption spreads, GPU sales are hitting record highs. NVIDIA’s data center revenue grew over 80% in 2023 to nearly $15 billion—directly reflecting the demand for AI computing power.
But here’s the twist: as each GPU generation gets more powerful, its power consumption and cooling requirements also rise. For example, the H100 GPU has about 30% higher TDP than the A100, meaning cooling systems must deal with more heat.
The result? The GPU cooling market is growing rapidly. Research firms predict the data center cooling market will exceed $20 billion by 2025, with a significant share devoted to GPU-specific cooling solutions.
2. How Do GPU Coolers Work?
To understand the importance of GPU cooling, we need to break down how it works. The primary goal is to transfer heat from the GPU chip to the surrounding environment, keeping the chip within a safe temperature range.
The Issue: High-performance GPUs can generate hundreds of watts of heat at full load. This heat needs to be removed quickly and efficiently.
Imagine a high-end AI GPU as a miniature hot plate, continuously pumping out heat. Without effective dissipation, the temperature quickly rises to dangerous levels.
The Risk: For every 10°C rise in temperature, electronic component failure rates double. Poor thermal management can cause premature failure in GPUs worth tens of thousands of dollars.
Even worse, GPU thermal density (heat per unit area) is increasing. As chips become smaller and more densely packed with transistors, more heat is generated in a smaller area—making heat removal harder.
The Solution: Modern GPU coolers use various techniques, mainly including:
Air Cooling
The most common method, made up of heatsinks and fans:
- Heatsinks: Made from aluminum or copper, designed to increase surface area and quickly release heat into the air.
- Heat Pipes: Copper tubes filled with fluid that use phase change (evaporation and condensation) to transfer heat efficiently.
- Fans: Move air through the heatsink, carrying heat away.
Liquid Cooling Systems
For high-performance AI GPUs, liquid cooling is more effective:
- Closed-Loop Liquid Cooling (AIO): Coolant circulates in a sealed system, absorbing GPU heat via a water block and releasing it via a radiator.
- Open-Loop Liquid Cooling: More advanced, often used in data centers, connects to a central cooling system.
- Immersion Cooling: Servers are submerged in non-conductive cooling fluids for maximum heat transfer efficiency.
GPU Cooling Technology Comparison |
---|
Cooling Method |
———————— |
Air Cooling |
Closed-Loop Liquid |
Open-Loop Liquid |
Immersion Cooling |
The Importance of Materials
The material used in a cooler plays a key role in performance. That’s why high-end coolers often use copper instead of aluminum.
Copper has a thermal conductivity of ~401 W/(m·K), versus aluminum’s 237 W/(m·K). Copper conducts heat faster but is heavier and more expensive. Many coolers use a hybrid design—copper at contact points, aluminum elsewhere—for a performance-cost balance.
Some cutting-edge solutions also use graphene or carbon nanotubes, offering elite thermal performance but at a high cost, currently limited to premium products.
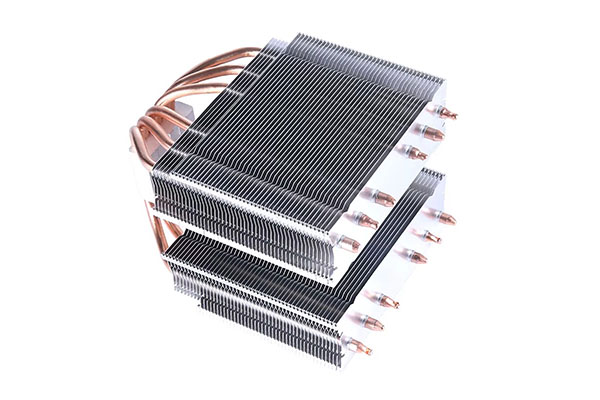
3. How Do Data Centers Tackle AI Server Cooling Challenges?
As enterprises accelerate AI infrastructure deployments, data centers are facing unprecedented cooling challenges. Traditional data centers were designed for rack power loads of 5–10kW, but modern AI server racks may demand 30–50kW or even more.
Here’s where things get interesting: data centers are undergoing a design revolution to handle these high-density AI computing environments.
Rise of Liquid Cooling
Traditional air cooling is becoming less viable due to the intense heat output of AI servers. Liquid cooling is quickly gaining traction:
- Direct-to-Chip Cooling: Coolant flows through cold plates attached directly to GPUs, transferring heat to the central cooling system. Can handle rack power up to 100kW.
- Immersion Cooling: Entire servers are submerged in dielectric cooling fluid. This method delivers the highest efficiency and supports over 100kW per rack while reducing cooling energy use by up to 95%.
But there’s more: these technologies not only improve thermal performance but also significantly cut energy use. Studies show liquid cooling can reduce data center cooling energy consumption by up to 40%—a massive win for companies focused on sustainability.
Thermal Management Software & AI Optimization
Modern data centers don’t rely solely on hardware. Intelligent software is becoming a major player:
- Predictive Thermal Management: AI algorithms forecast hot spots and intervene before problems arise.
- Dynamic Workload Distribution: Workloads are assigned based on heat distribution to avoid overheating clusters.
- Adaptive Cooling Control: Real-time temperature data is used to optimize cooling system behavior.
Data Center Cooling Comparison |
---|
طريقة |
———————- |
Traditional Air |
Heat Exchanger + Air |
Direct Liquid Cooling |
Immersion Cooling |
Note: PUE (Power Usage Effectiveness) indicates energy efficiency. Closer to 1 is better.
Sustainability & Heat Recovery
Here’s a key point: as energy use increases, sustainability becomes critical. Leading data centers are recovering waste heat as a resource:
- Heat Recovery Systems: Captured heat used for building heating or hot water.
- District Heating Integration: Heat sent to nearby communities for shared heating infrastructure.
- Combined Heat & Power (CHP): Recycled heat used for power generation to increase overall efficiency.
Example: Facebook’s data center in Denmark recovers nearly all its server heat and provides heating to over 6,900 households. This not only reduces environmental impact but adds economic value.
4. Market Trends in High-Performance GPU Cooling Solutions
With AI-driven computing on the rise, the GPU cooling market is undergoing explosive growth and innovation. Knowing these trends helps businesses better plan their AI infrastructure.
The Issue: Traditional cooling solutions can’t keep up with the thermal demands of next-gen AI GPUs.
Just five years ago, high-end GPUs had TDPs around 250–300W. Today, the NVIDIA H100 hits 700W—and future models may exceed 1,000W. This dramatic rise in power makes cooling a critical bottleneck for AI deployment.
The Risk: Insufficient cooling limits GPU performance and increases energy waste and operating costs. At scale, this translates into millions in extra expenses and carbon emissions.
Even more alarming: data center space and power are becoming scarce. Efficient cooling directly impacts how much computing power can fit per square meter—giving a major competitive edge.
The Solution: The market is responding with cutting-edge technologies and business models. Here are the top trends:
Mainstreaming of Liquid Cooling
Liquid cooling is going from niche to mainstream:
- Pre-Installed Liquid Systems: OEMs like Dell, HP, and Lenovo offer AI servers with integrated liquid cooling.
- Modular Liquid Cooling: Plug-and-play systems that integrate easily into existing data centers.
- Cold Plate Standardization: GPU vendors partner with cooling specialists to create universal interfaces.
Here’s the exciting part: liquid-cooled server shipments are projected to grow over 300% in the next five years, shifting this from “nice-to-have” to “must-have.”
Cooling-as-a-Service
As cooling systems become more complex, new business models are emerging:
- Managed Cooling Services: Specialist providers offer end-to-end cooling system design, installation, and maintenance.
- Performance-Based Contracts: Vendors guarantee specific thermal performance or pay penalties.
- Pay-as-You-Go Cooling: Companies pay based on actual cooling needs, not upfront infrastructure.
This model frees up capital for core business while ensuring top cooling performance.
GPU Cooling Market Trends |
---|
اتجاه |
—————————- |
Mainstream Liquid Cooling |
Cooling-as-a-Service |
Immersion Cooling Standardization |
Heat Recovery Integration |
Key Market Players
The GPU cooling space is attracting big investments and innovation:
- Traditional Cooling Vendors: Vertiv, Schneider Electric, Rittal are expanding data center portfolios.
- GPU Cooling Specialists: Asetek, CoolIT, Aquila focus on high-performance cooling.
- Emerging Innovators: LiquidStack, Green Revolution Cooling, Submer lead in immersion technology.
- GPU Makers: NVIDIA and AMD develop reference designs optimized for their own chips.
Worth noting: the market is consolidating, with large infrastructure firms acquiring cooling startups—like Vertiv buying Geist and E+I Engineering to boost its liquid cooling capabilities.
5. How to Choose the Right GPU Cooling System for AI Workloads
Selecting the right GPU cooling system for AI workloads is a complex task that requires evaluating multiple factors. Making the right decision impacts performance, reliability, and total cost of ownership.
The Issue: Different AI workloads have different cooling requirements. Choosing the wrong solution can lead to underperformance or wasted investment.
In AI, workloads vary widely—from short-term batch inference to weeks-long large-scale training. Each scenario demands a different cooling strategy.
The Risk: A poorly matched cooling system may cause thermal throttling or hardware failure. Over-engineered systems raise costs and complexity without added value.
What’s more, AI models and hardware evolve quickly—so today’s cooling needs may not fit tomorrow’s. That makes scalability a key concern in system selection.
The Solution: Take a structured approach to evaluate needs and select the right cooling technology:
Assessing Cooling Requirements
Start by evaluating your environment:
- Calculate Total Heat Load: Add up TDPs for all GPUs and other components.
- Consider Usage Patterns: Look at workload duration and intensity.
- Analyze Environmental Conditions: Include ambient temperature, humidity, air quality.
- Evaluate Space Constraints: Determine available physical layout and space limits.
A simple rule: add a 20–30% buffer to GPU TDPs to handle peak loads and future upgrades.
Cooling Technology Selection Framework
Match technology to deployment scale and workload:
GPU Cooling Selection Guide |
---|
Deployment Size |
——————— |
Single GPU/Workstation |
Small Servers (1–4 GPUs) |
Mid-size Cluster (5–20 GPUs) |
Large Data Center (>20 GPUs) |
Key Performance Metrics
Evaluate solutions based on:
- Thermal Resistance (°C/W): Lower is better for heat transfer.
- Noise Level (dBA): Critical for office or lab use.
- Energy Efficiency (PUE): Lower PUE means more efficient cooling.
- Reliability (MTBF): Especially for moving parts in liquid systems.
- Total Cost of Ownership (TCO): Includes hardware, energy, maintenance, and lifespan.
Don’t just look at upfront cost. For AI workloads, operating expenses often outweigh initial investment. A system that costs 20% more upfront could cut operating costs by 50% over five years.
Future Scalability
AI evolves fast. Choose systems that can grow with you:
- Modular Design: Makes scaling easier.
- Over-Provisioned Capacity: Leave 20–30% cooling headroom for future GPUs.
- Compatibility: Ensure system supports upcoming GPU models.
- Upgrade Path: Know how to expand to higher-capacity systems later.
That’s one reason many companies choose liquid cooling—it typically offers a clearer path to scale, whether by adding modules or moving up to immersion.
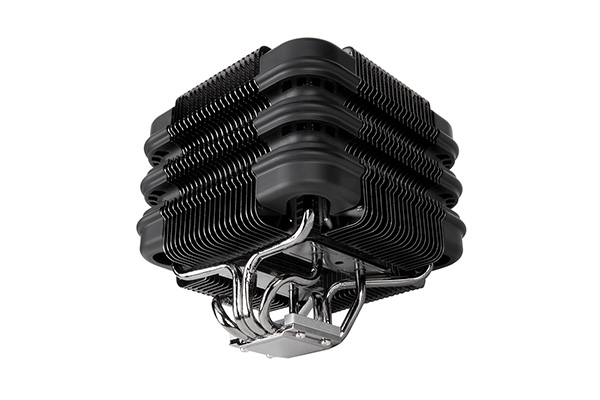
التعليمات
Q1: What is a GPU cooler?
A GPU cooler is a thermal system designed to keep the graphics processing unit (GPU) within safe operating temperatures. It removes heat via methods like air cooling, liquid cooling, or immersion, helping prevent overheating, performance drops, or hardware failure.
Q2: Why do AI servers need special cooling?
AI workloads—especially deep learning training and inference—push GPUs to run near full power for extended periods, generating far more heat than typical tasks. Modern AI GPUs can have TDPs of 300–700 watts and stay at high loads for days or weeks, requiring advanced cooling systems to maintain stability and extend hardware life.
Q3: What’s the difference between liquid and air cooling
Air cooling uses heatsinks and fans to push heat into the air. Liquid cooling uses fluids that have much higher heat capacity (about 4,000 times that of air) to absorb and transfer heat. Liquid systems—closed-loop, open-loop, or immersion—offer better cooling performance, lower noise, and more stable temperatures, but at higher cost and complexity.
Q4: How do data centers handle cooling for high-density AI servers?
They adopt multiple strategies:
- Advanced liquid cooling (direct-to-chip, immersion),
- Redesigning racks and airflow,
- AI-powered thermal management software,
- Heat recovery systems for building heating,
- Modular setups mixing standard and high-density zones.
These methods improve efficiency and support power densities of 30–50kW or more per rack.
Q5: How do I choose the right GPU cooler for my AI workloads?
Consider:
- Workload type (intensity, duration),
- Deployment scale (workstation to data center),
- Environmental factors (ambient temp, space),
- Performance targets (temps, noise),
- Total cost of ownership (hardware, energy, maintenance),
- Future scalability (GPU upgrades, modularity).
For small setups, air or AIO liquid cooling may suffice. For large-scale AI clusters, direct liquid or immersion cooling is usually required.