Introduction
The artificial intelligence revolution has created unprecedented thermal management challenges for data centers and high-performance computing environments. As AI models grow increasingly complex, the GPUs and specialized accelerators powering them generate heat levels that push traditional cooling solutions beyond their limits. This comprehensive article explores innovative cooling approaches that are reshaping how we manage thermal challenges in AI infrastructure.
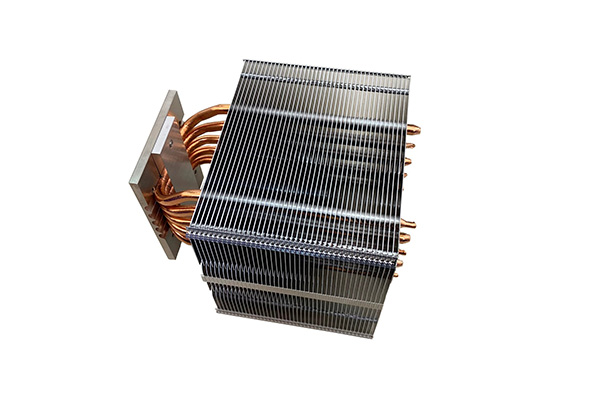
The Thermal Crisis in AI Computing
The exponential growth in AI computing power has created thermal challenges that traditional cooling approaches simply cannot address effectively.
Problem: Modern AI accelerators generate unprecedented heat densities that exceed the capabilities of conventional cooling solutions.
Today’s high-performance AI GPUs like NVIDIA’s H100 or AMD’s MI300 can generate thermal loads exceeding 700 watts per device—more than triple what gaming GPUs produced just a few years ago. When deployed in dense configurations with multiple accelerators per server, rack-level heat generation can reach 50-100kW, creating cooling challenges that conventional approaches struggle to address.
Aggravation: The trend toward higher power consumption in AI accelerators shows no signs of slowing, with next-generation devices potentially exceeding 1000W.
Further complicating matters, AI workloads typically maintain these devices at near 100% utilization for extended periods—sometimes weeks or months—creating sustained thermal loads fundamentally different from traditional computing workloads with their variable utilization patterns.
Solution: A new generation of innovative cooling technologies is emerging to address these unprecedented thermal challenges:
The Evolution of AI Accelerator Thermal Output
Understanding the dramatic increase in cooling requirements:
- Historical Perspective on GPU Thermal Design Power (TDP):
- 2010: NVIDIA Fermi (GTX 480) – 250W
- 2015: NVIDIA Maxwell (Titan X) – 250W
- 2018: NVIDIA Volta (V100) – 300W
- 2020: NVIDIA Ampere (A100) – 400W
- 2022: NVIDIA Hopper (H100) – 700W
- 2024: Next-gen AI accelerators – 800-1000W+ (projected)
- Factors Driving Increased Power Consumption:
- Larger die sizes and transistor counts
- Higher clock speeds and voltage requirements
- Increased memory bandwidth and capacity
- More specialized compute units
- Performance prioritization over efficiency
- Unique Thermal Characteristics of AI Workloads:
- Sustained maximum utilization
- Extended run times (days to weeks)
- Minimal idle or low-power periods
- Consistent rather than variable thermal output
- Limited opportunity for thermal recovery
Here’s what makes this fascinating: The thermal output of AI accelerators has grown at approximately 2.5x the rate predicted by Moore’s Law. While traditional computing hardware typically sees 15-20% power increases per generation, AI accelerators have experienced 50-100% TDP increases across recent generations. This accelerated thermal evolution reflects a fundamental shift in design philosophy, where performance is prioritized even at the cost of significantly higher power consumption and thermal output.
The Limitations of Traditional Cooling
Understanding why conventional approaches fall short:
- Air Cooling Constraints:
- Practical limit of 350-400W per device
- Density limitations (4-6 high-power GPUs per server)
- Airflow restrictions in dense environments
- Fan power consumption and noise issues
- Temperature uniformity challenges
- Basic Liquid Cooling Challenges:
- Cold plate design limitations
- Flow distribution inefficiencies
- Heat exchanger capacity constraints
- Implementation complexity
- Scaling difficulties in large deployments
- Facility-Level Restrictions:
- Traditional data center cooling capacity limits
- Power distribution constraints
- Space utilization inefficiencies
- Water availability and quality issues
- Heat rejection capacity limitations
But here’s an interesting phenomenon: The cooling challenge for AI accelerators represents a fundamental inversion of traditional computing heat patterns. In traditional systems, CPUs typically generate 60-70% of the total heat, with GPUs as secondary contributors. In modern AI systems, accelerators often account for 70-80% of the total thermal output, with CPUs reduced to a secondary heat source despite their own substantial thermal output. This inversion requires a complete rethinking of system thermal design, with cooling resources allocated proportionally to this new heat distribution.
The Economic Imperative for Innovation
Understanding the business drivers behind cooling innovation:
- Performance and Capability Impact:
- Thermal throttling reducing computational capacity by 10-30%
- Extended training times increasing time-to-results
- Inconsistent inference performance affecting service quality
- Reduced hardware utilization efficiency
- Opportunity costs of delayed AI implementation
- Infrastructure Cost Implications:
- Density constraints limiting computational capacity
- Facility limitations restricting expansion
- Energy efficiency challenges increasing operational costs
- Hardware lifespan reduction from thermal stress
- Capital investment inefficiencies
- Competitive and Strategic Factors:
- AI capabilities increasingly tied to cooling capacity
- Time-to-market advantages from superior cooling
- Research and development velocity dependencies
- Computational capacity as competitive differentiator
- Strategic flexibility requirements
Impact of Cooling Quality on AI Infrastructure Performance
Cooling Quality | Temperature Range | Performance Impact | Reliability Impact | Density Capability | Economic Consequence |
---|---|---|---|---|---|
Inadequate | 85-95°C+ | Severe throttling, 30-50% performance loss | 2-3x higher failure rate | 4-6 GPUs per server | 40-60% effective capacity loss |
Borderline | 75-85°C | Intermittent throttling, 10-30% performance loss | 1.5-2x higher failure rate | 6-8 GPUs per server | 20-40% effective capacity loss |
Adequate | 65-75°C | Minimal throttling, 0-10% performance impact | Baseline failure rate | 8-10 GPUs per server | 0-10% effective capacity loss |
Optimal | 45-65°C | Full performance, potential for overclocking | 0.5-0.7x failure rate | 10-16 GPUs per server | 10-20% effective capacity gain |
Premium | <45°C | Maximum performance, sustained boost clocks | 0.3-0.5x failure rate | 16+ GPUs per server | 20-30% effective capacity gain |
Ready for the fascinating part? Research indicates that inadequate cooling can reduce the effective computational capacity of AI infrastructure by 15-40%, essentially negating much of the performance advantage of premium GPU hardware. This “thermal tax” means that organizations may be realizing only 60-85% of their theoretical computing capacity due to cooling limitations, fundamentally changing the economics of AI infrastructure. When combined with the reliability impact, the total cost of inadequate cooling can exceed the price premium of advanced cooling solutions within the first year of operation for high-utilization AI systems.
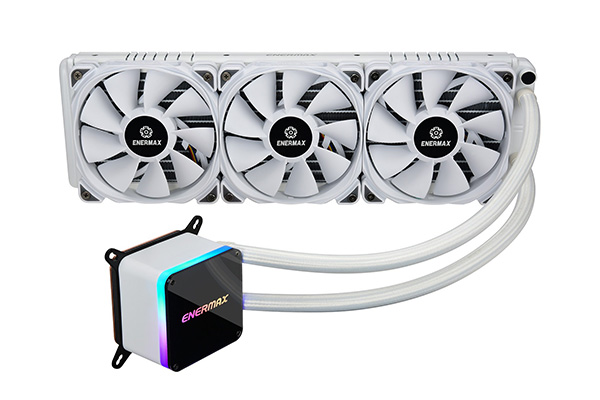
Beyond Traditional Air Cooling
While air cooling remains the most common approach for general computing, AI accelerators require more advanced solutions to address their extreme thermal challenges.
Problem: Traditional air cooling approaches are fundamentally limited in their ability to address the thermal density of modern AI accelerators.
The physics of air as a heat transfer medium creates inherent limitations that make it increasingly impractical for cooling devices exceeding 400W, particularly in dense multi-accelerator configurations.
Aggravation: The density requirements of AI deployments often exceed what conventional air cooling can support, creating a need for alternative approaches.
Further complicating matters, the energy consumption of high-performance fans increases dramatically as thermal loads rise, creating efficiency challenges that compound the direct cooling limitations.
Solution: Advanced air cooling innovations and hybrid approaches are extending the practical limits of air-based thermal management for AI accelerators:
Advanced Air Cooling Techniques
Pushing the boundaries of air cooling performance:
- Heat Pipe and Vapor Chamber Advancements:
- Ultra-thin heat pipe designs (0.6-1.2mm)
- Sintered powder wick implementations
- Multi-pipe arrays (8-12 pipes typical)
- Vapor chamber base plates replacing solid copper
- Custom geometries for GPU dies
- Advanced Material Applications:
- Graphene-enhanced heat pipes
- Synthetic diamond heat spreaders
- Carbon nanotube thermal interfaces
- Phase change thermal materials
- Composite fin structures
- Airflow Optimization Techniques:
- Computational fluid dynamics optimization
- Variable fin density arrangements
- Ducted and channeled designs
- Turbulence-inducing geometries
- System-level airflow integration
Here’s what makes this fascinating: The thermal conductivity of advanced cooling materials has improved dramatically in recent years. While traditional copper heat pipes offer thermal conductivity around 3,000-4,000 W/m·K, new graphene-enhanced heat pipes can achieve 6,000-8,000 W/m·K—a 2x improvement that directly translates to better cooling performance. Similarly, synthetic diamond heat spreaders offer 5-10x better thermal conductivity than copper, though at significantly higher cost. These material advances are enabling air cooling to handle thermal loads that would have required liquid cooling just a few years ago.
Hybrid Cooling Approaches
Bridging the gap between air and liquid cooling:
- Assisted Air Cooling Solutions:
- Thermoelectric cooling assistance
- Heat pipe to remote radiator designs
- Refrigerant-assisted systems
- Synthetic jet augmentation
- Phase change material integration
- Targeted Liquid Cooling:
- GPU-only liquid cooling
- Air cooling for secondary components
- Simplified implementation
- Focused cooling resource allocation
- Transitional approach for gradual adoption
- Split System Implementations:
- External radiator configurations
- Rear door heat exchanger integration
- Top-of-rack cooling solutions
- Facility water connection options
- Modular cooling expansion capability
But here’s an interesting phenomenon: The relationship between airflow and cooling performance follows a non-linear curve with diminishing returns. Doubling airflow typically improves cooling by only 30-40% due to the physics of convective heat transfer. This non-linearity creates practical limits for air cooling that cannot be overcome simply by adding more or larger fans. Instead, the most effective advanced air cooling solutions focus on optimizing the entire thermal path from die to ambient air, with innovations at each stage of heat transfer working together to maximize overall performance.
Containment and Isolation Strategies
Optimizing the environment for cooling efficiency:
- Hot/Cold Aisle Containment:
- Complete physical separation
- Airflow management optimization
- Temperature differential maximization
- Recirculation prevention
- Efficiency improvement of 20-40%
- Rack-Level Containment:
- Enclosed rack implementations
- Dedicated cooling per rack
- Thermal isolation from environment
- Density optimization
- Deployment flexibility enhancement
- Server-Level Thermal Design:
- Internal airflow optimization
- Component placement for thermal efficiency
- Impedance matching with system fans
- Pressure management techniques
- Temperature-based dynamic control
Advanced Air Cooling Technologies for AI Accelerators
Technology | Cooling Capacity | Key Advantages | Limitations | Best Applications | Implementation Complexity |
---|---|---|---|---|---|
Vapor Chamber | Up to 400W | Excellent heat spreading, low profile | Limited distance heat transfer | High-density servers, limited space | Moderate |
Graphene-Enhanced | Up to 450W | Superior thermal conductivity | Manufacturing complexity, cost | Premium solutions, thermal constraints | High |
Synthetic Diamond | Up to 500W | Ultimate thermal conductivity | Extremely high cost | Research, specialized applications | Very High |
Thermoelectric Assisted | Up to 450W | Active cooling capability | Power consumption, complexity | Hotspot management, premium solutions | High |
Hybrid Air/Liquid | Up to 600W | Balanced performance and simplicity | Partial benefits, compromise solution | Transitional deployments, mixed workloads | Moderate |
Case Studies and Real-World Performance
Examining practical implementations of advanced air cooling:
- High-Density Server Implementations:
- 4U server with 8x 350W GPUs
- Vapor chamber and heat pipe combination
- Custom ducting and airflow management
- Temperature results and performance impact
- Density and efficiency achievements
- Research Computing Clusters:
- University deployment case study
- Budget-constrained implementation
- Performance optimization techniques
- Thermal monitoring and management
- Lessons learned and best practices
- Edge AI Deployments:
- Space-constrained implementations
- Passive and semi-passive designs
- Environmental challenge adaptations
- Reliability in variable conditions
- Total cost of ownership considerations
Ready for the fascinating part? The most advanced air cooling solutions are now implementing “intelligent cooling” features that dynamically adapt to changing thermal conditions. These systems use embedded sensors, variable fan curves, and even adjustable heat pipe characteristics to optimize cooling based on real-time workload and environmental factors. Some cutting-edge designs can shift their cooling capacity between multiple GPUs based on utilization, effectively “load balancing” thermal management resources. This adaptive approach can improve cooling efficiency by 15-25% compared to static designs, representing a fundamental shift from passive to active thermal management at the component level.
Advanced Liquid Cooling Architectures
Liquid cooling has emerged as the primary solution for addressing the thermal challenges of high-performance AI accelerators.
Problem: The thermal output of modern AI accelerators exceeds the practical capabilities of even advanced air cooling, necessitating more effective heat transfer methods.
With thermal densities exceeding 0.5-1.0 W/mm² and total package power reaching 400-700+To save on context only part of this file has been shown to you. You should retry this tool after you have searched inside the file with grep -n
in order to find the line numbers of what you are looking for.
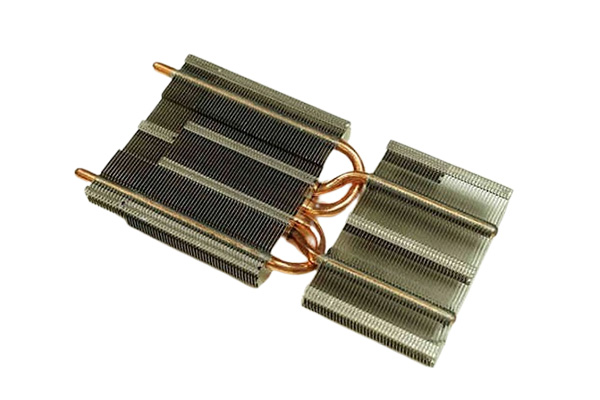
FAQ Section
Q1: What are AI accelerators, and why do they need specialized cooling?
AI accelerators, such as GPUs and TPUs, are specialized hardware designed to accelerate AI computations. These devices perform complex tasks much faster than traditional CPUs, generating significant heat in the process. As AI workloads become more demanding, traditional cooling methods like air cooling often fall short. Specialized cooling solutions, such as liquid cooling or phase-change cooling, are necessary to manage the heat efficiently, ensuring optimal performance and preventing overheating, which can degrade hardware lifespan and performance.
Q2: What makes innovative cooling solutions different from traditional cooling?
Traditional cooling solutions typically rely on air coolers or basic liquid cooling systems. Innovative solutions go beyond these methods by incorporating advanced technologies like immersion cooling, loop cooling, or even micro-channel cooling systems. These approaches can handle higher thermal loads, reduce energy consumption, and maintain stable temperatures more effectively than traditional air or basic liquid cooling. By using cutting-edge materials and techniques, these systems are designed to optimize the performance of AI accelerators, especially in high-demand environments.
Q3: How does immersion cooling work for AI accelerators?
Immersion cooling involves submerging the AI accelerators directly into a non-conductive liquid that absorbs and dissipates heat. This technique is highly efficient because it allows for direct heat transfer from the hardware to the liquid, which then carries the heat away to a cooling system. It eliminates the need for traditional fans or heat sinks, reducing the physical space required and improving cooling efficiency. Immersion cooling is particularly useful for AI data centers that run large-scale models and require consistent, efficient heat management.
Q4: Are there any downsides to using innovative cooling systems for AI accelerators?
While innovative cooling solutions offer significant advantages, they also come with challenges. Systems like immersion cooling require specialized infrastructure, which can be costly to implement. Moreover, these advanced systems may require more maintenance and monitoring compared to traditional cooling solutions. However, the benefits of enhanced performance, energy efficiency, and long-term hardware reliability often outweigh these downsides, especially in high-performance computing environments where optimal cooling is crucial for success.
Q5: How can AI companies benefit from adopting innovative cooling solutions?
AI companies can benefit from adopting innovative cooling solutions in several ways. These advanced systems enable better heat management, which can lead to higher operational efficiency and faster processing times for AI tasks. By maintaining optimal temperatures, AI accelerators are less likely to experience thermal throttling, ensuring consistent performance during intensive computations. Additionally, innovative cooling systems help extend the lifespan of expensive AI hardware, reduce downtime, and lower overall energy consumption, leading to significant cost savings in the long term.