소개
The artificial intelligence revolution has fundamentally transformed data center cooling requirements, creating unprecedented thermal management challenges that demand innovative solutions. As organizations deploy increasingly powerful GPUs and specialized AI accelerators, traditional data center cooling approaches are proving inadequate to address the extreme heat densities and sustained thermal loads generated by AI workloads. This comprehensive article explores the design and implementation of cooling infrastructure specifically optimized for AI-focused data centers.
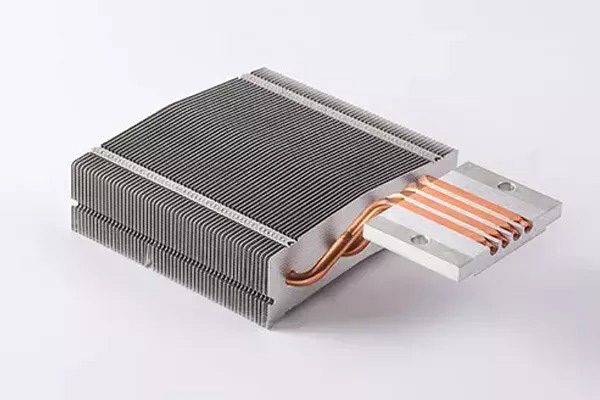
The AI-Driven Transformation of Data Center Cooling
The rise of artificial intelligence has catalyzed a fundamental shift in data center thermal management requirements and approaches.
Problem: Traditional data center cooling infrastructure was designed for IT loads with substantially different thermal characteristics than modern AI workloads.
Conventional data centers typically supported 5-10kW per rack with relatively uniform heat distribution and variable utilization patterns—a far cry from the 30-100kW+ per rack, GPU-concentrated heat generation, and sustained maximum utilization characteristic of AI infrastructure.
Aggravation: The rapid growth of AI deployments is creating cooling demands that exceed the capabilities of existing data center infrastructure.
Further complicating matters, many organizations are attempting to deploy AI infrastructure in facilities originally designed for general-purpose computing, creating significant cooling challenges that must be addressed through retrofits or new construction.
해결책: A new generation of data center cooling infrastructure has evolved specifically to address the thermal challenges of AI workloads:
The Evolution of Data Center Cooling
Understanding how cooling infrastructure has adapted to changing requirements:
- Traditional Data Center Cooling (Pre-2010):
- Raised floor air distribution
- Computer Room Air Conditioning (CRAC) units
- Hot/cold aisle configuration
- 5-10kW per rack capacity
- 2.0+ Power Usage Effectiveness (PUE)
- High-Performance Computing Era (2010-2018):
- In-row cooling supplementation
- Rear door heat exchangers
- Containment systems implementation
- 10-25kW per rack capacity
- 1.3-1.8 PUE typical
- AI-Optimized Cooling (2018-Present):
- Direct liquid cooling integration
- Facility water distribution systems
- Immersion cooling implementations
- 30-100kW+ per rack capacity
- 1.1-1.4 PUE achievable
Here’s what makes this fascinating: The thermal density increase in data centers has accelerated dramatically with AI adoption. While traditional data centers saw density increases of approximately 10-15% annually, AI-focused facilities are experiencing 30-50% annual growth in per-rack thermal density. This accelerated thermal evolution is forcing a compressed innovation cycle in cooling infrastructure, with technologies that would normally take 10+ years to become mainstream being adopted within 3-5 years due to necessity.
The Unique Thermal Profile of AI Workloads
Understanding what makes AI different from a cooling perspective:
- Heat Density Characteristics:
- Traditional servers: 250-500W per device
- AI accelerators: 400-700W+ per device
- Traditional racks: 5-10kW total
- AI racks: 30-100kW+ total
- Heat concentration in GPU/accelerator components
- Utilization and Workload Patterns:
- Sustained maximum utilization (90-100%)
- Extended run times (days to weeks)
- Minimal idle or low-power periods
- Consistent rather than variable thermal output
- Limited opportunity for thermal recovery
- Scaling and Growth Trajectories:
- Rapid deployment expansion
- Increasing model sizes driving higher power
- Growing accelerator counts per system
- Higher power devices in each generation
- Compounding thermal management challenges
But here’s an interesting phenomenon: The thermal profile of AI infrastructure represents a fundamental inversion of traditional computing heat patterns. In traditional data centers, compute load was distributed relatively evenly across servers, with CPUs as the primary heat source. In AI data centers, heat generation is highly concentrated in GPU/accelerator-dense servers, which may represent only 20-30% of the total server count but generate 70-80% of the total heat load. This concentration creates “thermal hotspots” that require targeted cooling approaches rather than uniform facility-wide solutions.
The Economic Imperative for Cooling Innovation
Understanding the business drivers behind cooling infrastructure investment:
- Performance and Capability Impact:
- Thermal throttling reducing computational capacity by 10-30%
- Extended training times increasing time-to-market
- Inconsistent inference performance affecting service quality
- Reduced hardware utilization efficiency
- Opportunity costs of delayed AI implementation
- Infrastructure Cost Implications:
- Density constraints limiting computational capacity
- Facility limitations restricting expansion
- Energy efficiency challenges increasing operational costs
- Hardware lifespan reduction from thermal stress
- Capital investment inefficiencies
- Competitive and Strategic Factors:
- AI capabilities increasingly tied to cooling capacity
- Time-to-market advantages from superior cooling
- Research and development velocity dependencies
- Computational capacity as competitive differentiator
- Strategic flexibility requirements
The Evolution of Data Center Cooling for AI Workloads
Era | Typical Density | Primary Cooling Approach | PUE Range | Key Challenges | Typical Applications |
---|---|---|---|---|---|
Traditional (Pre-2010) | 5-10kW/rack | Raised floor air cooling | 2.0-3.0 | Efficiency, hotspots | General computing, web services |
High-Performance (2010-2018) | 10-25kW/rack | In-row cooling, containment | 1.3-1.8 | Density, air limitations | HPC, early AI, financial modeling |
Early AI (2018-2020) | 20-40kW/rack | Rear door HX, direct liquid | 1.2-1.5 | Retrofit challenges, scaling | AI research, model development |
Modern AI (2020-Present) | 30-100kW+/rack | Direct liquid, immersion | 1.1-1.4 | Facility integration, standards | Production AI, large model training |
Next-Gen AI (Emerging) | 100kW+/rack | Two-phase, integrated cooling | 1.05-1.2 | Power delivery, heat rejection | Frontier AI, specialized infrastructure |
Ready for the fascinating part? Research indicates that inadequate cooling infrastructure can reduce the effective computational capacity of AI systems by 15-40%, essentially negating much of the performance advantage of premium GPU hardware. This “thermal tax” means that organizations may be realizing only 60-85% of their theoretical computing capacity due to cooling limitations, fundamentally changing the economics of AI infrastructure. When combined with the reliability impact, the total cost of inadequate cooling can exceed the price premium of advanced cooling infrastructure within the first year of operation for high-utilization AI systems.
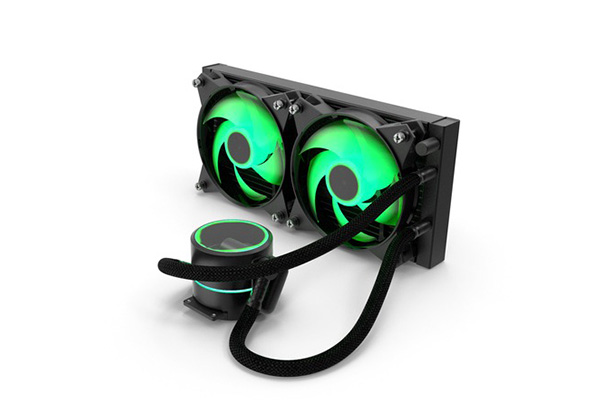
Assessing Cooling Requirements for AI Workloads
Accurately determining cooling requirements is the essential first step in designing effective infrastructure for AI deployments.
Problem: Many organizations struggle to accurately assess the cooling requirements for AI infrastructure, leading to either inadequate cooling or costly overprovisioning.
The unique thermal characteristics of AI workloads, combined with rapid evolution in hardware capabilities and deployment models, create significant challenges in requirements planning.
Aggravation: The trend toward higher power consumption in AI accelerators shows no signs of abating, making future-proofing particularly challenging.
Further complicating matters, many organizations lack experience with advanced cooling technologies, creating knowledge gaps that hinder effective requirements assessment and planning.
해결책: A structured, comprehensive approach to cooling requirements assessment enables more accurate infrastructure planning:
Thermal Load Calculation Methodology
Developing accurate cooling requirement estimates:
- Component-Level Power Assessment:
- GPU/accelerator thermal design power (TDP)
- CPU power consumption
- Memory and storage thermal contribution
- Power delivery component heat generation
- Networking and peripheral heat output
- System-Level Integration Factors:
- Multi-GPU configurations
- Server chassis thermal characteristics
- Airflow restrictions and impedance
- Component proximity effects
- System-level power management
- Workload-Specific Considerations:
- Utilization patterns and duration
- Application-specific power profiles
- Development vs. production workloads
- Batch vs. real-time processing
- Seasonal and cyclical variations
Here’s what makes this fascinating: The relationship between theoretical TDP and actual cooling requirements varies significantly based on workload characteristics. While traditional IT planning often uses a 60-70% “diversity factor” (assuming not all components will operate at maximum power simultaneously), AI workloads typically require 90-100% of theoretical maximum due to sustained utilization patterns. This fundamental difference in planning assumptions is a primary reason why many early AI deployments experienced cooling challenges despite following established data center design practices.
Density and Distribution Planning
Optimizing the spatial arrangement of thermal load:
- Rack-Level Density Considerations:
- Maximum kW per rack determination
- Vertical distribution within racks
- Airflow and cooling medium delivery
- Structural and weight limitations
- Power delivery constraints
- Row and Pod Configuration:
- Hot/cold aisle implementation
- Containment system requirements
- In-row cooling supplementation
- Rack arrangement optimization
- Maintenance access requirements
- Facility-Wide Distribution Strategies:
- High-density zones vs. uniform distribution
- Cooling resource allocation
- Future expansion accommodation
- Phased deployment planning
- Retrofit vs. new construction considerations
But here’s an interesting phenomenon: The optimal density for AI infrastructure follows a non-linear cost curve that varies based on cooling technology and facility characteristics. For air-cooled deployments, cost-efficiency typically peaks around 15-25kW per rack, with higher densities creating exponentially increasing cooling challenges. For liquid-cooled deployments, the optimal density is often 40-60kW per rack, with immersion cooling enabling cost-effective densities of 80-100kW+ per rack. This variable density optimization means that “maximum possible density” is rarely the most economical approach, with the optimal point depending on specific technology choices and facility constraints.
Future-Proofing and Scaling Considerations
Planning for growth and evolution:
- Hardware Evolution Trajectories:
- Next-generation accelerator TDP projections
- Power density evolution trends
- Form factor and mounting changes
- Cooling interface standardization
- Emerging technology compatibility
- Deployment Growth Forecasting:
- Expansion timeline projections
- Scaling pattern predictions
- Technology refresh cycles
- Workload evolution expectations
- Budget and investment planning
- Flexibility and Adaptability Requirements:
- Technology transition accommodation
- Cooling capacity modulation
- Infrastructure upgrade pathways
- Vendor and technology lock-in avoidance
- Risk mitigation approaches
AI Workload Cooling Requirement Assessment Factors
요인 | Traditional Approach | AI-Optimized Approach | Impact on Requirements | Assessment Methodology |
---|---|---|---|---|
Utilization Pattern | 60-70% of maximum | 90-100% of maximum | 30-40% higher cooling need | Workload profiling, benchmarking |
Duration | Variable, with idle periods | Sustained maximum for days/weeks | Elimination of recovery periods | Training time analysis, scheduling review |
Component Focus | Balanced CPU/storage/memory | GPU/accelerator dominated | Targeted cooling requirements | Hardware inventory, power monitoring |
Density | 5-15kW per rack | 30-100kW+ per rack | Facility-level cooling limitations | Deployment planning, technology selection |
Growth Trajectory | Gradual, predictable | Rapid, step-function increases | Future-proofing challenges | AI roadmap analysis, industry benchmarking |
Cooling Technology Selection Criteria
Matching requirements to appropriate cooling approaches:
- Performance Requirements Analysis:
- Temperature targets and stability needs
- Density and capacity requirements
- Efficiency and sustainability goals
- Reliability and redundancy expectations
- Acoustic and environmental constraints
- Facility Constraint Assessment:
- Existing infrastructure compatibility
- Space and floor loading limitations
- Water availability and quality
- Power distribution capabilities
- Heat rejection capacity
- Operational Considerations:
- Staff expertise and capabilities
- Maintenance requirements and procedures
- Monitoring and management systems
- Serviceability and access needs
- Lifecycle and replacement planning
Ready for the fascinating part? The most sophisticated organizations are implementing “cooling portfolio strategies” rather than standardizing on a single approach. By deploying different cooling technologies for different workloads and deployment scenarios, these organizations optimize both performance and economics across their AI infrastructure. Some have found that a carefully balanced portfolio approach can improve overall price-performance by 20-40% compared to homogeneous deployments, while simultaneously providing greater flexibility to adapt to evolving requirements. This portfolio approach represents a fundamental shift from viewing cooling as a standardized infrastructure component to treating it as a strategic resource that should be optimized for specific use cases.
Facility-Level Cooling Infrastructure
The foundation of effective AI cooling begins with properly designed facility infrastructure that can support the extreme thermal demands of modern accelerators.
Problem: Traditional data center cooling infrastructure lacks the capacity, efficiency, and flexibility required for AI workloads.
Conventional raised floor air cooling systeTo save on context only part of this file has been shown to you. You should retry this tool after you have searched inside the file with grep -n
in order to find the line numbers of what you are looking for.
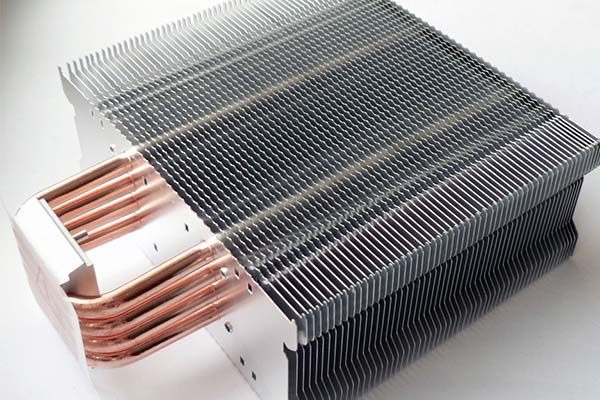
자주 묻는 질문
Q1: What is Data Center Cooling Infrastructure for AI Workloads?
Data center cooling infrastructure for AI workloads refers to the systems and technologies designed to maintain optimal operating temperatures for AI systems housed in data centers. AI workloads, particularly those involving machine learning, deep learning, and other intensive computational tasks, generate a significant amount of heat. Effective cooling infrastructure ensures that these systems perform efficiently without overheating. It includes cooling methods like air conditioning, liquid cooling, and specialized cooling units designed for high-performance computing equipment.
Q2: Why is Cooling Infrastructure Critical for AI Workloads?
Cooling infrastructure is essential for AI workloads because these tasks often require high-performance processors, GPUs, and other hardware that generate substantial heat during operation. Without proper cooling, the temperature of these components can rise beyond acceptable limits, leading to throttling, reduced performance, or even hardware damage. Cooling systems help mitigate these risks, maintaining the longevity of the equipment and ensuring continuous, optimal performance for AI applications, which are often time-sensitive and resource-intensive.
Q3: What Are the Common Cooling Solutions Used in AI Workloads?
There are several cooling solutions commonly used in data centers for AI workloads:
- Air Cooling: Involves cooling the components with fans that circulate air over heat sinks. It’s cost-effective but might not be efficient enough for extremely high-performance systems.
- Liquid Cooling: Involves circulating a coolant through pipes attached to critical components, offering better thermal efficiency than air cooling. This method is especially useful for AI servers with dense hardware configurations.
- Immersion Cooling: Involves submerging components in a thermally conductive liquid that directly cools the hardware. This method is gaining popularity in high-performance data centers that need to manage significant heat output from AI workloads.
Q4: What Are the Challenges in Implementing Cooling Systems for AI Workloads?
Implementing effective cooling systems for AI workloads presents several challenges:
- Heat Density: AI workloads often rely on dense hardware configurations, which can lead to a high concentration of heat in specific areas.
- Energy Consumption: Advanced cooling systems, especially liquid and immersion cooling, require a significant amount of energy, which can increase operational costs.
- Space Constraints: Many data centers are space-constrained, making it difficult to implement large-scale cooling systems without reducing the area available for computing equipment.
Q5: How Do You Choose the Right Cooling System for AI Workloads?
Choosing the right cooling system for AI workloads depends on several factors, including the scale of the AI infrastructure, the density of the hardware, energy efficiency goals, and available space. For smaller AI setups, air cooling or traditional liquid cooling systems may suffice. However, for large-scale AI operations, especially those requiring high-density servers, liquid cooling or immersion cooling systems are more effective. It’s also essential to consider the total cost of ownership, including energy consumption, maintenance, and equipment lifespan, to make the most informed choice.