A Deep Dive into the Latest Cooling Technologies
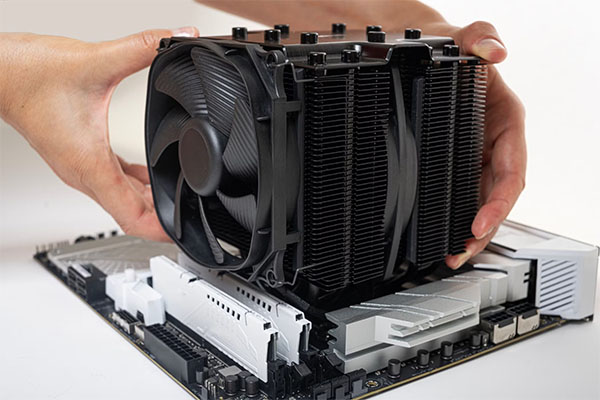
High-power GPU coolers are essential components of modern AI training infrastructure. They are purpose-built to handle the extreme heat output generated by artificial intelligence workloads. As AI models continue to scale in complexity and size, GPU cooling technology is rapidly evolving to meet the demands. In this article, we’ll explore the latest advancements, innovative solutions, and how these technologies are enabling the future of AI training.
Table of Content
- Why Does AI Training Present Unprecedented Challenges for GPU Cooling?
- What Are the Breakthroughs in Modern High-Power GPU Cooling Technologies?
- How Liquid Cooling Is Reshaping AI Training Infrastructure
- Cost-Benefit Analysis of High-Power GPU Cooling Solutions
- What Are the Future Trends in GPU Cooling Technologies?
- คำถามที่พบบ่อย
1. Why Does AI Training Present Unprecedented Challenges for GPU Cooling?
The rise of AI—especially deep learning—is pushing computing hardware into a new era marked by massive compute density and power consumption, particularly across GPU accelerators.
The Issue: Modern AI training workloads place extreme thermal demands on GPU cooling systems.
Imagine this: training a large language model (LLM) might require hundreds or thousands of GPUs operating continuously for weeks or months. Each GPU is running at or near full power, constantly generating high heat output.
Key Differences from Traditional Computing Workloads:
- Sustained High Load: AI training pushes GPUs near 100% utilization instead of intermittent use.
- Extended Run Times: Models may train continuously for weeks.
- High-Density Deployment: GPUs are densely packed to optimize training, compounding thermal strain.
The Risk: Overheating leads to performance throttling, shortens hardware life, and may cause irreversible damage.
Impact Overview:
คุณสมบัติ | Traditional Computing | AI Training | Impact |
---|---|---|---|
Usage Pattern | Intermittent/Variable | Sustained Load | No cooling breaks |
Runtime Duration | Hours | Days/Weeks | Long-term thermal stability |
Power Density | Medium | สูงมาก | Requires high-efficiency cooling |
Thermal Sensitivity | ปานกลาง | สูง | Precise thermal control needed |
Deployment Density | Low/Medium | High/Very High | Hotspot management challenges |
Thermal Design Power (TDP) of Next-Gen AI GPUs
GPU Model | TDP Rating |
---|---|
NVIDIA H100 | Up to 700W |
AMD Instinct MI300 | ~750W |
Intel Gaudi 3 | >600W (expected) |
Note: Actual peak power may exceed rated TDPs during intensive AI tasks.
Experts predict next-gen AI accelerators may surpass 1,000W TDP, pushing the limits of current thermal management technologies.
2. What Are the Breakthroughs in Modern High-Power GPU Cooling Technologies?
The Issue: Traditional cooling methods can’t cope with the latest GPU heat outputs.
The Challenge: Increasing power density creates hot spots on chips. These can be 10–15°C hotter than average temperature zones—critical failure points if unmanaged.
Latest Innovations:
วัสดุขั้นสูง
Material/Tech | Thermal Conductivity | Key Advantage |
---|---|---|
Graphene Heatsinks | Up to 5,000 W/(m·K) | Up to 60% better heat transfer |
Phase Change Materials | ~200–300 J/g | Stabilizes thermal spikes |
Carbon Nanotube TIMs | ~30% lower interface resistance | Enhanced thermal interface conductivity |
Microfluidic Cooling
คุณสมบัติ | คำอธิบาย |
---|---|
3D Printed Microchannels | Custom cooling flow to GPU hot zones |
Two-Phase Cooling | Liquid-to-vapor transfer increases thermal efficiency |
Smart Flow Control | Sensor-based regulation for real-time cooling optimization |
Comparative Cooling Tech Overview
เทคโนโลยี | Thermal Efficiency | ความซับซ้อน | ค่าใช้จ่าย | กรณีการใช้งานที่ดีที่สุด |
---|---|---|---|---|
Graphene Heatsinks | สูงมาก | Medium | สูง | Workstations/Servers |
Phase Change | สูง | ต่ำ | Medium | Variable temp environments |
Microfluidics | สูงมาก | สูงมาก | สูงมาก | Dense data centers |
Jet Cooling | Extremely High | สูง | สูง | Ultra-high power density GPUs |
Immersion Cooling | สูง | Medium | สูง | Large-scale deployment |
Jet Cooling
Advanced jet systems can handle 500 W/cm²—future-ready for next-gen AI accelerators.
3. How Liquid Cooling Is Reshaping AI Training Infrastructure
Liquid cooling is becoming a mainstream solution for AI infrastructure, fundamentally reshaping how data centers are designed and operated.
The Issue: Air has reached its cooling limits in high-density AI environments.
Metric | Air Cooling | Water Cooling |
---|---|---|
Heat Capacity | ~1 J/(g·K) | ~4.18 J/(g·K) |
Density | ต่ำ | ~830x higher |
The Challenge: Rack power densities are jumping from 5–10kW to 50–100kW+. Air cooling requires impractical space and airflow volumes at this level.
Direct-to-Chip (D2C) Cooling
- Cold Plate Designs:
- Microchannel structures
- Jet-impingement targeting hot spots
- Hybrid materials (e.g., copper + graphene)
- Coolant Distribution Units (CDUs):
- Smart CDUs monitor flow, pressure, and temps
- Redundant systems ensure uptime
- Smart Control Systems:
- AI-powered thermal load prediction
- Real-time GPU temperature feedback
Immersion Cooling Revolution
Cooling Type | คำอธิบาย |
---|---|
Single-Phase | Non-conductive fluid, natural or forced convection |
Two-Phase | Fluid evaporates at GPU surface, condenses, and recycles |
Modular Systems | Pre-built immersion tanks with scalable architecture |
Liquid Cooling Impact on AI Infrastructure
Aspect | Air Cooling | Direct Liquid | Immersion |
---|---|---|---|
Rack Power Density | 5–15 kW | 30–60 kW | 100+ kW |
PUE (Efficiency) | 1.4–1.8 | 1.1–1.3 | 1.02–1.1 |
Thermal Uniformity | Poor | ดี | ยอดเยี่ยม |
Noise | สูง | ต่ำ | Very Low |
การซ่อมบำรุง | ต่ำ | Medium | Med–High |
CapEx | ต่ำ | Med–High | สูง |
OpEx | สูง | Medium | ต่ำ |
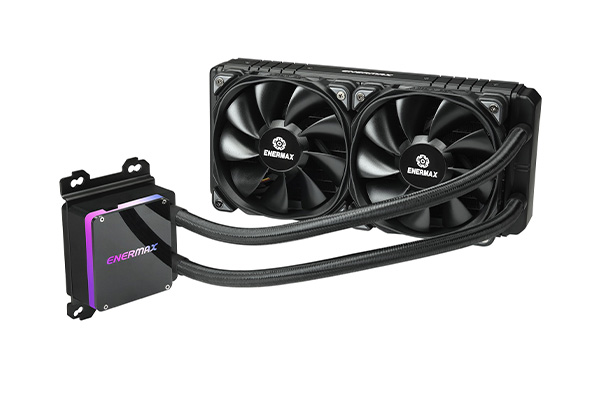
4. Cost-Benefit Analysis of High-Power GPU Cooling Solutions
The Issue: High initial costs often deter adoption of advanced cooling systems.
The Risk: Focusing only on upfront costs leads to missed opportunities in performance, reliability, and long-term savings.
Initial vs. Operational Cost Breakdown
Cost Type | High-End Air | Direct Liquid | Immersion Cooling |
---|---|---|---|
Initial Hardware | $200–500/GPU | $500–1,500/GPU | $1,000–2,500/GPU |
Installation | ต่ำ | Medium | สูง |
Energy (5 years) | $250,000 | $150,000 | $125,000 |
Maintenance (5 yrs) | $25,000 | $35,000 | $30,000 |
Space (5 yrs) | $100,000 | $60,000 | $40,000 |
Downtime (Est.) | $50,000 | $20,000 | $15,000 |
5-Year TCO (100 GPUs) | $465,000 | $375,000 | $410,000 |
Note: Actual figures depend on energy pricing, workload utilization, and facility specs.
ROI from Performance & Reliability
- Performance Uplift:
- 5–15% AI throughput gain from thermal throttling avoidance
- Shorter training time = faster model deployment
- Extended GPU Lifespan:
- Lower temps double component life expectancy
- Upgrade cycle extended from 2–3 years to 4–5 years
- Improved Uptime:
- Lower failure rates
- Fewer training interruptions
Scalability & Future-Proofing
ผลประโยชน์ | Impact |
---|---|
Power Density Headroom | Ready for next-gen GPUs with 1,000W+ TDP |
Space Optimization | Smaller footprint per compute unit |
Regulatory Compliance | Supports green goals, energy incentives possible |
5. What Are the Future Trends in GPU Cooling Technologies?
The Issue: Current cooling tech may fall short for 1,500W+ AI accelerators projected in 5–7 years.
Emerging Technologies
เทคโนโลยี | ETA to Market | Efficiency Gain | Advantage | ท้าทาย |
---|---|---|---|---|
Supercritical CO₂ | 2–4 years | 30–40% | High efficiency, eco-friendly | Complex high-pressure setup |
Magnetic Cooling | 3–5 years | 20–30% | No refrigerants, high efficiency | Cost, scalability |
Nanofluid Cooling | Now–2 years | 15–40% | Retrofits existing systems | Long-term stability |
On-Chip Liquid Channels | 2–3 years | 50–70% | Ultra-short thermal path | Manufacturing complexity |
3D Stack Cooling | 3–5 years | N/A* | Enables dense chip packaging | Thermal path innovation |
Not a direct efficiency gain but enables architectural breakthroughs.
Integrated Thermal Design
Future systems will embed cooling from the ground up:
- On-Die Microchannels:
- Liquid channels inside chip package
- 3D Chip Stack Cooling:
- Vertical heat dissipation solutions like heat pipes and microjets
- System-Level Thermal Management:
- AI-controlled full-stack temperature regulation
Sustainable Cooling & Heat Recovery
- High-Temp Recovery:
- Organic Rankine Cycle systems convert waste heat into electricity
- Tiered Utilization:
- High-temp for power, mid-temp for industry, low-temp for heating
- Seasonal Heat Storage:
- Stores summer heat for winter reuse
Exciting Insight: Future data centers may reach near-perfect PUE (~1.0) while powering local communities with excess clean energy.
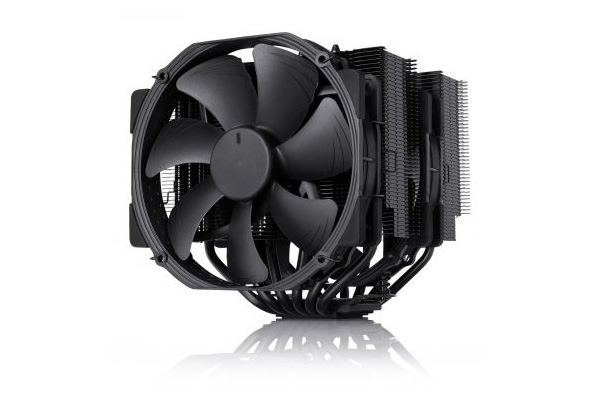
คำถามที่พบบ่อย
Q1: What is a high-power GPU cooler?
A high-power GPU cooler is designed to manage the extreme heat output of modern AI GPUs. Compared to traditional coolers, these use advanced materials and designs—like microchannel plates, PCM, and high-conductivity composites—to handle loads of 300–700W or more.
Q2: Why is AI training more thermally demanding than regular workloads?
AI training keeps GPUs at near 100% load for days or weeks, often in tightly packed configurations. This results in:
- Constant, high heat output
- Thermal hotspots
- Sensitivity to even minor temperature fluctuations
Q3: What’s the difference between air and liquid GPU cooling
Aspect | Air Cooling | Liquid Cooling |
---|---|---|
Medium | Air (low thermal capacity) | Liquid (4,000x thermal capacity) |
ผลงาน | Moderate, temperature 70–85°C | 15–25°C lower than air systems |
Noise | Higher, needs large fans | Quieter, more efficient transfer |
Reliability | Fewer moving parts | Pumps, valves (more complex) |
ค่าใช้จ่าย | Lower upfront | Higher CapEx, lower OpEx over time |
Q4: How cost-effective are high-power GPU cooling solutions?
The cost-effectiveness of high-power GPU cooling solutions should be evaluated from a total cost of ownership (TCO) perspective, not just the initial investment. Although the initial cost of advanced cooling systems (such as direct liquid cooling or immersion cooling) may be 2-3 times higher than traditional air cooling systems, they generally provide significant long-term economic advantages: in terms of energy costs, efficient heat dissipation can reduce cooling energy consumption by 30-50%; in terms of performance, better temperature control can improve GPU computing efficiency by 5-15% and accelerate training completion; in terms of hardware life, a stable low-temperature environment can extend the life of the GPU and reduce the frequency of replacement; in terms of space utilization, efficient heat dissipation allows for higher-density deployment and reduces data center space requirements. In a typical deployment cycle of 5 years, the total cost of ownership of an efficient cooling solution is typically 20-30% lower than that of a traditional system.
Q5: What are the main development trends of GPU cooling technology in the future?
The main development trends of GPU cooling technology in the future include: emerging cooling technologies, such as supercritical CO₂ cooling, magnetic cooling, and nanofluid cooling, which are expected to provide 30-70% higher cooling efficiency than traditional methods; integrated heat dissipation design, integrating the cooling system directly into the chip package or even the silicon wafer, greatly reducing the heat conduction path; dedicated heat dissipation solutions for 3D stacked chips to solve the unique challenges of the next generation of computing architecture; system-level thermal management, using AI algorithms to optimize the entire thermal path from chip to facility; sustainable development and heat recovery technology, converting data center waste heat into useful energy for power generation or district heating. These trends together point to a future where heat dissipation will no longer be an independent after-the-fact component, but a core consideration integrated into the design of computing systems from the beginning.