Giới thiệu
The artificial intelligence revolution has created unprecedented thermal management challenges for graphics processing units (GPUs) and specialized AI accelerators. As AI models grow increasingly complex and computationally intensive, the heat generated by these high-performance processors has reached levels that demand innovative cooling solutions. This comprehensive article explores the most effective thermal management strategies for AI GPUs, providing practical guidance for organizations seeking to optimize performance, reliability, and efficiency.
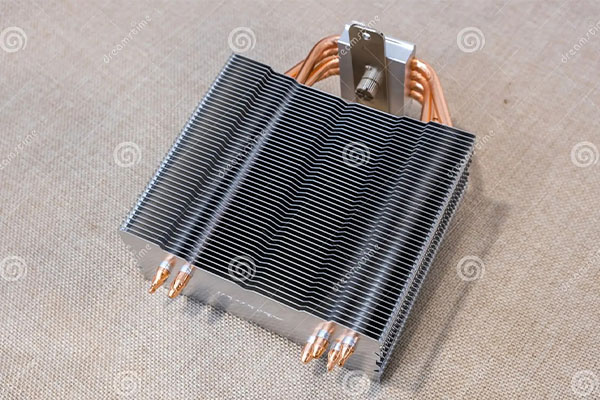
Understanding the Thermal Challenge of AI GPUs
The thermal characteristics of modern AI GPUs create unique cooling challenges that require specialized solutions.
Vấn đề: Modern AI GPUs generate unprecedented levels of heat that exceed the capabilities of traditional cooling approaches.
Today’s high-performance AI GPUs like NVIDIA’s H100 or AMD’s MI300 can generate thermal loads exceeding 700 watts per device—more than triple what gaming GPUs produced just a few years ago. This dramatic increase in power consumption creates thermal challenges that conventional approaches struggle to address effectively.
Aggravation: The sustained nature of AI workloads creates thermal conditions fundamentally different from other computing applications.
Further complicating matters, AI workloads typically maintain GPUs at near 100% utilization for extended periods—sometimes weeks or months—creating sustained thermal loads without the variable utilization patterns and recovery periods characteristic of other computing workloads.
Giải pháp: Effective thermal management for AI GPUs requires a comprehensive understanding of their unique characteristics and the application of specialized cooling technologies:
The Thermal Profile of Modern AI GPUs
Understanding the unique heat generation characteristics:
- Power Consumption Characteristics:
- Modern AI GPUs: 400-700W+ per device
- Gaming GPUs: 250-450W per device
- Traditional server GPUs: 150-300W per device
- Sustained vs. variable power draw
- Thermal Design Power (TDP) vs. actual consumption
- Heat Density Considerations:
- Modern AI GPUs: 0.5-1.0 W/mm²
- Gaming GPUs: 0.3-0.5 W/mm²
- Traditional CPUs: 0.2-0.3 W/mm²
- Die size and packaging effects
- Hotspot formation and management
- Thermal Distribution Patterns:
- GPU die heat generation
- Memory subsystem thermal output
- VRM and power delivery heat
- PCB and passive component heating
- System-level thermal interactions
Here’s what makes this fascinating: The thermal output of AI GPUs has grown at approximately 2.5x the rate predicted by Moore’s Law. While traditional computing hardware typically sees 15-20% power increases per generation, AI accelerators have experienced 50-100% TDP increases across recent generations. This accelerated thermal evolution reflects a fundamental shift in design philosophy, where performance is prioritized even at the cost of significantly higher power consumption and thermal output.
The Impact of AI Workloads on Thermal Conditions
Understanding how AI applications affect thermal management:
- Utilization Patterns:
- Sustained maximum utilization (90-100%)
- Extended run times (days to weeks)
- Minimal idle or low-power periods
- Consistent rather than variable thermal output
- Limited opportunity for thermal recovery
- Computational Characteristics:
- Tensor core intensive operations
- High memory bandwidth utilization
- Parallel processing across all compute units
- Power-optimized operation for performance
- Workload-specific thermal patterns
- Deployment Scenarios:
- Multi-GPU configurations (4-16+ per server)
- High-density rack deployments
- Varied environmental conditions
- 24/7 operation requirements
- Scale-out cluster implementations
But here’s an interesting phenomenon: The thermal profile of AI workloads creates a fundamentally different cooling challenge compared to other high-performance computing applications. While gaming workloads might generate high heat for hours at a time with variable intensity, AI training can maintain maximum thermal output for days or weeks without interruption. This sustained thermal load means that cooling systems must be designed not just for peak performance but for continuous operation at that peak, with minimal thermal cycling or recovery periods.
Performance and Reliability Implications
Understanding the critical relationship between thermal management and GPU effectiveness:
- Thermal Throttling Effects:
- Clock speed reduction under thermal stress
- Performance degradation of 10-30% during throttling
- Training time extension and cost implications
- Inconsistent inference performance
- Reduced return on hardware investment
- Hardware Reliability Considerations:
- Each 10°C increase approximately doubles failure rates
- Thermal cycling creates mechanical stress
- Memory errors increase at elevated temperatures
- Power delivery components vulnerable to thermal stress
- Economic impact of hardware failures and replacements
- Operational Stability Requirements:
- AI workloads require consistent performance
- Reproducibility challenges with variable thermal conditions
- Production deployment stability expectations
- 24/7 operation for many AI systems
- Business continuity considerations
Impact of Thermal Management Quality on AI GPU Performance and Reliability
Cooling Quality | GPU Temperature Range | Performance Impact | Reliability Impact | Operational Impact | Economic Consequence |
---|---|---|---|---|---|
Inadequate | 85-95°C+ | Severe throttling, 30-50% performance loss | 2-3x higher failure rate | Unstable, frequent interruptions | 40-60% effective capacity loss |
Borderline | 75-85°C | Intermittent throttling, 10-30% performance loss | 1.5-2x higher failure rate | Periodic issues, inconsistent performance | 20-40% effective capacity loss |
Đủ | 65-75°C | Minimal throttling, 0-10% performance impact | Baseline failure rate | Generally stable with occasional issues | 0-10% effective capacity loss |
Optimal | 45-65°C | Full performance, potential for overclocking | 0.5-0.7x failure rate | Consistent, reliable operation | 10-20% effective capacity gain |
Premium | <45°C | Maximum performance, sustained boost clocks | 0.3-0.5x failure rate | Exceptional stability and longevity | 20-30% effective capacity gain |
Ready for the fascinating part? Research indicates that inadequate thermal management can reduce the effective computational capacity of AI infrastructure by 15-40%, essentially negating much of the performance advantage of premium GPU hardware. This “thermal tax” means that organizations may be realizing only 60-85% of their theoretical computing capacity due to cooling limitations, fundamentally changing the economics of AI infrastructure. When combined with the reliability impact, the total cost of inadequate cooling can exceed the price premium of advanced cooling solutions within the first year of operation for high-utilization AI systems.
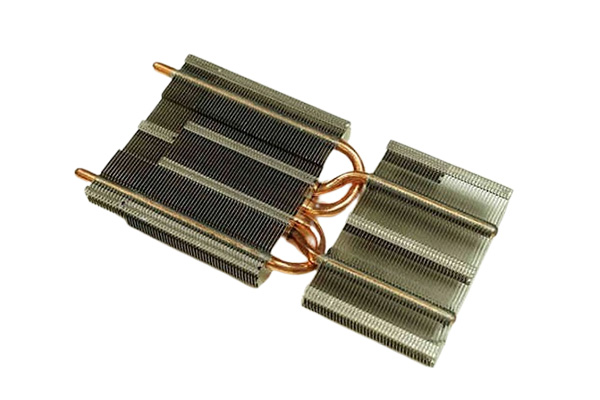
Advanced Air Cooling Techniques
While air cooling faces fundamental physical limitations for the highest-power AI GPUs, significant innovations continue to extend its practical capabilities.
Vấn đề: Traditional air cooling approaches are insufficient for modern AI GPUs, but liquid cooling may be impractical in many environments.
Many organizations face facility constraints, operational limitations, or budget restrictions that make liquid cooling challenging to implement, creating demand for enhanced air cooling solutions that can bridge the gap.
Aggravation: The sustained high utilization of AI workloads creates thermal challenges beyond what traditional air cooling was designed to handle.
Further complicating matters, the density requirements of AI deployments often exceed what conventional air cooling can support, creating a need for solutions that enable higher density while remaining air-based.
Giải pháp: Advanced air cooling innovations are extending the practical limits of air-based thermal management for AI GPUs:
Heat Pipe and Vapor Chamber Technology
Leveraging phase change for enhanced heat transfer:
- Heat Pipe Operating Principles:
- Sealed tube containing working fluid
- Evaporation at heat source
- Vapor transport to cooler regions
- Condensation and return via capillary action
- Effective thermal conductivity of 3,000-4,000 W/m·K
- Advanced Heat Pipe Implementations:
- Ultra-thin heat pipe designs (0.6-1.2mm)
- Sintered powder wick structures
- Multi-pipe arrays (8-12 pipes typical)
- Custom shapes and configurations
- Application-specific optimization
- Vapor Chamber Technology:
- Flat, planar heat spreading
- Two-dimensional thermal distribution
- Direct die contact implementation
- Integration with traditional heat pipes
- Large surface area coverage
Here’s what makes this fascinating: The thermal conductivity of advanced heat transfer devices creates a virtual “thermal superconductivity” effect. While copper has a thermal conductivity of approximately 400 W/m·K, heat pipes can achieve effective thermal conductivity of 3,000-4,000 W/m·K—nearly 10x better than solid copper. This dramatic improvement enables much more efficient heat transport from the GPU die to the cooling fins, significantly enhancing overall cooling performance without changing the fundamental air cooling approach.
Advanced Materials and Manufacturing
Pushing the boundaries of air cooling performance:
- Advanced Material Applications:
- Graphene-enhanced heat pipes (6,000-8,000 W/m·K)
- Synthetic diamond heat spreaders (1,000-2,200 W/m·K)
- Carbon nanotube thermal interfaces
- Phase change thermal materials
- Composite fin structures
- Manufacturing Technique Advancements:
- Additive manufacturing for complex geometries
- Skived fin production for increased density
- Vapor chamber integration techniques
- Bonding method improvements
- Precision machining advancements
- Surface Area Optimization:
- Fin density optimization
- Surface treatment for improved convection
- Turbulence-inducing geometries
- Stacked and folded fin structures
- Weight and space optimization
But here’s an interesting phenomenon: The relationship between airflow and cooling performance follows a non-linear curve with diminishing returns. Doubling airflow typically improves cooling by only 30-40% due to the physics of convective heat transfer. This non-linearity creates practical limits for air cooling that cannot be overcome simply by adding more or larger fans. Instead, the most effective advanced air cooling solutions focus on optimizing the entire thermal path from die to ambient air, with innovations at each stage of heat transfer working together to maximize overall performance.
Airflow Optimization Techniques
Maximizing the cooling potential of air:
- Fan Technology Advancements:
- High static pressure fan designs
- Fluid dynamic bearing implementations
- Variable speed control algorithms
- Noise optimization techniques
- Efficiency and reliability improvements
- Airflow Path Engineering:
- Ducted and channeled designs
- Impedance matching with system fans
- Turbulence management features
- Pressure drop optimization
- Recirculation prevention
- System-Level Airflow Integration:
- Server chassis airflow optimization
- Rack-level air management
- Containment system implementation
- Pressure management techniques
- Temperature-based dynamic control
Advanced Air Cooling Technologies for AI GPUs
Công nghệ | Cooling Capacity | Key Advantages | Limitations | Cost Premium | Best Applications |
---|---|---|---|---|---|
Traditional Heat Pipes | Up to 300W | Proven reliability, cost-effective | Limited capacity | Baseline | Entry-level AI, mixed workloads |
Vapor Chamber | Up to 400W | Excellent heat spreading, low profile | Limited distance heat transfer | 30-50% | High-density servers, limited space |
Advanced Heat Pipes | Up to 350W | Flexible implementation, proven reliability | Point-to-point transfer limitations | 10-30% | General purpose, cost-sensitive |
Graphene-Enhanced | Up to 450W | Superior thermal conductivity | Manufacturing complexity, cost | 50-100% | Premium solutions, thermal constraints |
Synthetic Diamond | Up to 500W | Ultimate thermal conductivity | Extremely high cost | 200-300%+ | Research, specialized applications |
Hybrid and Assisted Air Cooling
Bridging the gap between traditional air and liquid cooling:
- Thermoelectric Cooling Applications:
- Peltier effect utilization
- Targeted hotspot management
- Hybrid thermoelectric/air systems
- Efficiency optimization approaches
- Control system integration
- Phase Change Material Integration:
- Thermal energy storage capability
- Peak load management
- Passive temperature regulation
- Integration with active cooling
- Thermal buffer implementation
- Assisted Air Cooling Approaches:
- Heat pipe to remote radiator designs
- Refrigerant-assisted systems
- Synthetic jet augmentation
- Hybrid air/liquid solutions
- Transitional cooling technologies
Ready for the fascinating part? The most advanced air cooling solutions are now implementing “intelligent cooling” features that dynamically adapt to changing thermal conditions. These systems use embedded sensors, variable fan curves, and even adjustable heat pipe characteristics to optimize cooling based on real-time workload and environmental factors. Some cutting-edge designs can shift their cooling capacity between multiple GPUs based on utilization, effectively “load balancing” thermal management resources. This adaptive approach can improve cooling efficiency by 15-25% compared to static designs, representing a fundamental shift from passive to active thermal management at the component level.
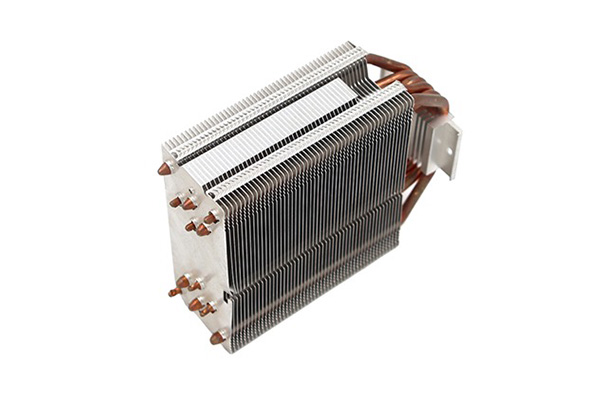
Liquid Cooling Solutions for AI GPUs
As AI GPU thermal output continues to increase, liquid cooling has become essential for high-performance deployments.
Vấn đề: The thermal output of modern AI GPUs exceeds the practical capabilities of even advanced air cooling, necessitating more effective heat transfer methods.
With thermal densities exceeding 0.5-1.0 W/mm² and total package power reaching 400-700+ watts, modern AI GPUs generate heat beyond what air cooling can effectively dissipate, particularly in dense deployments.
Aggravation: The trend toward higher GPU power consumptTo save on context only part of this file has been shown to you. You should retry this tool after you have searched inside the file with grep -n
in order to find the line numbers of what you are looking for.
Câu hỏi thường gặp
Q1: What are thermal management strategies for AI GPUs?
Thermal management strategies for AI GPUs focus on controlling and reducing the heat generated during intensive computations. These strategies ensure that GPUs maintain optimal performance and prevent overheating, which can cause throttling or damage to the hardware. Common strategies include using advanced cooling solutions like liquid cooling, enhancing airflow within the system, using high-performance thermal pastes, and implementing GPU thermal throttling to protect components when temperatures rise.
Q2: Why is thermal management crucial for AI GPUs?
Thermal management is critical for AI GPUs because these components generate significant heat when performing complex calculations, especially during AI model training and inference tasks. Without proper cooling, the GPU can overheat, leading to thermal throttling, where the GPU reduces its performance to prevent damage. Effective thermal management ensures stable performance, extends the lifespan of the GPU, and prevents system crashes or slowdowns due to excessive temperatures.
Q3: What are the most common cooling solutions for AI GPUs?
The most common cooling solutions for AI GPUs are air cooling and liquid cooling. Air cooling uses heat sinks and fans to dissipate heat from the GPU, providing a straightforward and cost-effective solution. However, for high-performance tasks, liquid cooling offers superior heat dissipation by circulating a coolant through tubes and a radiator. Liquid cooling is often preferred in AI applications because it can handle the higher thermal output of modern GPUs more efficiently, ensuring better performance and lower temperatures.
Q4: How can airflow be optimized for better thermal management?
Optimizing airflow is essential for improving thermal management in AI GPU setups. Proper airflow ensures that cool air is directed towards the GPU and other critical components while hot air is efficiently expelled. To achieve this, users should position fans strategically within the case to create positive airflow. Intake fans should be placed at the front, and exhaust fans should be placed at the rear or top of the case. Additionally, ensuring that the cables and components do not obstruct airflow can further enhance cooling performance.
Q5: Can thermal management strategies improve the lifespan of AI GPUs?
Yes, thermal management strategies can significantly improve the lifespan of AI GPUs. By preventing the GPU from overheating and ensuring that temperatures remain within safe limits, these strategies reduce the likelihood of thermal stress, which can degrade hardware over time. Efficient cooling solutions prevent premature failure of the GPU’s internal components, such as the memory and transistors, thereby extending the lifespan and maintaining high performance for a longer period. Proper thermal management not only protects the hardware but also maximizes the ROI on expensive AI GPU investments.